How do data lakes differ from data warehouses and hubs?
Many data analytics experts consider data hubs, data lakes and data warehouses as interchangeable alternatives. However, each of these structures has a specific role and a different primary purpose. Find out what the differences between them are and why it's best for your business to be able to combine them.
Different structure, different focus
Data is becoming increasingly diverse - as are its sources and the ways you can handle it. New requirements are continually emerging in the business and beyond, for which a traditional architecture based on central data collection for predefined use is no longer sufficient - or at least not the only and most efficient way.
The challenge for data analytics and architecture leaders is to provide a modern data management infrastructure that supports flexibility, diversity of data needs, and connectivity. But this often cannot be done without a combination of different approaches to data organization and processing. Yet some still focus on meeting all needs through a single architectural pattern - and choose between a traditional enterprise warehouse, a modern data lake or a data hub as if they were exclusively competing approaches.
However, each of these structures can serve different goals. Yet all three are key areas of enterprise investment, so it is important to focus on them and clarify both their similarities and fundamental differences.
Data warehouse
A data warehouse is a collection of data in which two or more disparate data sources can be combined into an integrated, time-varying information management strategy. "It is therefore primarily used to consolidate and evaluate data, usually with a fixed structure and a given purpose," says Milan Bartoš, Data Management Manager at Trask.
Data warehouses generally support well-known, predefined and repeatable analytical needs that can be scaled across many users in the enterprise. They thus best meet the requirements for medium to highly consistent semantics. They also typically support a fairly fixed processing strategy (SQL-oriented access to a central physical data repository) and offer a suitable solution for complex queries, high levels of concurrency, and stringent performance requirements. However, they alone do not allow a company to be more agile. "Simply put, data warehouses are for the long haul; you have to know in advance what data and what you'll want from it in, say, a year," Bartoš describes one of the weaknesses of a data warehouse.
Data lake
Data lakes collect raw data, or data in its native form with limited transformation and quality assurance, and events captured from disparate source systems. They typically support data preparation, exploratory analysis, and data science activities - potentially across a wide range of subjects and constituents. As a result, they also support highly variable semantics, a generic set of analytic use cases, and a variety of processing styles and approaches, including data discovery, machine learning, and intensive batch computing.
"The lakes blend everything together and everyone can pull out what they need right now. They are very efficient at consuming data that matches the current desires of people in the company. For example, if someone needs a report on a given day, they can have instant output from it," Milan Bartoš simplifies. "However, there is a lack of consolidation compared to data warehouses. You can't get to some data, or it's very laborious."
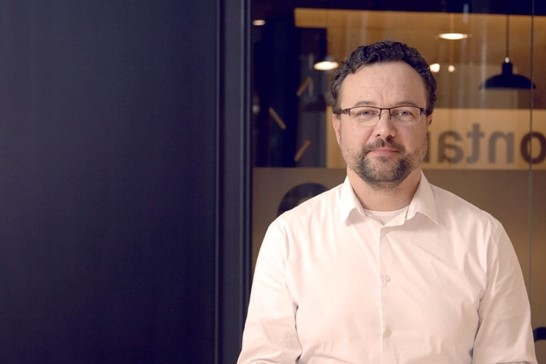
Data hub
Unlike datawarehouses and data lakes, data hubs are not focused on the analytical use ofdata. Rather, a data hub is an architectural pattern that enables seamless dataflow and management. It interconnects data producers and data consumers,applying data management controls and common models to enable efficient datasharing.
Data nodesthen focus primarily on managing consistent semantics. They can support anumber of use cases - most often of an operational nature, for example, inproviding master data to business applications and processes. They can alsosupport a range of processing strategies by allowing the choice of datapersistence techniques, integration styles and access methods.
What to think about when comparing warehouse,lake and hub
Datawarehouses and data lakes are similar. Both structures provide an endpoint forcollecting transactional, detailed data, specifically to support analyticaltasks.
What doesthis mean? That you can run different types of analysis on them, access thedata they hold, and support the analytical needs of the business. In addition,lakes and warehouses can include governance controls, for example throughmonitoring and resolving incoming data quality issues. However, they supportgovernance in a more reactive and "downstream" manner.
Data hubsare quite different in comparison, as they typically do not store detailed datafor extended periods of time. They do not serve as repositories on whichanalytical tasks are performed, rather they are places for data brokering andsharing. The individual data nodes in the hubs then enable the flow of data inthe enterprise by connecting the systems and processes producing the data withthe systems and processes consuming the data. A data hub can then also be usedto connect enterprise applications to a data warehouse or data lake.
Althoughthe optimal use of each structure separately may be quite different, thedistinction between the two may blur depending on the design approaches andtechnology choices. Especially when both are used simultaneously. As a result,the data warehouse, data lake and data hub can be combined very well to worktogether in an efficient architecture.
How to combine these structures effectively?
Data Hub Centric model
An exampleof an effective solution is a combination in which data is delivered to theanalytic structures (data warehouses and data lakes) through a data hub thatacts as an intermediary and control point (see Figure below). More and moreenterprises are adopting a data hub architecture as the focal point for sharingand managing all critical data across the enterprise. This includes theprovisioning of data from operational systems to analytical structures such asdata warehouses and data lakes.

A logicaldata warehouse can be supported through the federation of data that resides inthe data lake and data warehouse. The different characteristics of datawarehouses and data lakes mean that both models are increasingly required tosupport the diverse analytical needs of the modern enterprise. The combinationof data warehouse and data lake capabilities represents a common type oflogical data warehouse.
Data Lake Centric model
The secondmodel involves placing the data lake directly behind the source systems. Asanalytical tasks become more diverse, organizations are increasingly looking toleverage the insights gained in the data lake and scale them for broader, repeatable use across the enterprise through the data warehouse.
Likewise,the ability to provide structured analytics results from the data warehouse tocustomers, suppliers and others is also increasing in demand. Data hubs can bean effective place to manage and deliver these needs.
Theinsights gained from data lake operations can be provided to the data warehouse(for recurring and scalable consumption) and the data used can be provideddirectly from the data lake to external consumers via a data hub (see Figurebelow). We can leverage this data (and increase the value provided) formultiple different constituents - both inside and outside the enterprise.

A keyelement of a modern data management infrastructure is therefore the ability tothink dynamically - that is, the ability to evolve architectural patterns overtime, enable new connections and support new use cases. Data and architectureteams should therefore regularly review requirements and decide how to respondto them, according to Milan Bartoš.
Youshould consider the following options:
- Combiningdifferent technologies (e.g., data lakes and data warehouses) to collect andanalyze data to ensure faster turnaround of business requests.
- Combiningdifferent technology locations (on-premise and cloud) to ensure efficientmanagement.
- Use ofuniversal data transfer tools or data interconnects.
- Use ofdynamic and distributed data storage.
For example, ŠKODA AUTO uses a data lake and a data warehouse as parts of acomprehensive data management solution. Interestingly, the data lake is locatedwithin the ŠKODA infrastructure, while the new data warehouse is already in thecloud. And we as Trask are very happy to be there, says Milan Bartoš,manager of Trask's Data Management team.